While artificial intelligence (AI) may be intimidating, Alison Wagonfeld, VP Marketing, Google Cloud, and David Edelman, Senior Lecturer of Business Administration at Harvard Business School and former F50 CMO, discuss how it can make a meaningful impact on the customer journey, and help you build to scale. They touch on all things AI, machine learning (ML), automation, and the influence on the customer journey. With inherent biases in data, it can lead to biased results; however, by standardizing on a set of agreed-upon AI principles and asking the right questions, you can help lead your teams to better high-impact outcomes.
Here is what they had to say during the Emerging Leader Forum and how AI could influence your team, talent, and future.
Overview:
- Personalized Advertising, Done Well
- Quick Refresher on AI and ML
- How to Deliver a Personalized Customer Experience Using AI and ML
- Business Problems AI and ML is Suited to Solve
- Applying AI and Optimizing Teams for Digital Transformation
- Three Helpful Tips for Using Data and AI
- Managing Inherent Biases in Data
Personalized Advertising, Done Well
David Edelman: My fascination with AI started just before I joined Aetna, about six years ago. My town in Massachusetts began to give incentives for people to put solar panels on their houses, and we got bombarded with various advertisements from solar panel companies.
One promotion came through the mail saying, “Edelman family, at your specific address, we believe you can save 25% on your electricity bills. Click on this website to find out how.” The URL included was personalized with my home address, so I typed it in and immediately saw an image of my home through Google Earth with solar panels superimposed on my roof. This organization figured out how many solar panels could fit, and based on the orientation of my house and the analyzed tree cover, they calculated how much electricity I could generate.
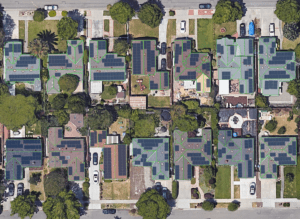
How SunPower puts solar on your roof with AI Platform (Photo Credit: Google Cloud)
Taking it a step further, they included information they brought in from Zillow about the square footage of my house to estimate how much our home’s energy was from electricity and gas. They calculated all this information using AI and ML to show how much electricity I consumed and what I would save by installing solar panels.
I was impressed, so in one click, I talked to a representative through video consultation; he had all my information accessible, and everything snowballed from there. It was an incredible experience, and I got the solar panels. This one ad started my curiosity about how to use AI capabilities to simplify something that could be incredibly complex.
Personalized Advertising, the Break Down
Alison Wagonfeld: To break down what happened in David’s excellent example, as marketers, we know everything starts with customer insights, but he also referenced the use of data. The solar panel company pulled data from multiple sources, using a spectrum of core analytics and AI to generate insights. When you have such a large body of data, it would be impossible for humans to analyze it, so we rely on AI and ML to analyze data and train it to look for specific insights.
Quick Refresher on AI and ML
By Alison Wagonfield
Artificial Intelligence: A program that can sense, reason, act, and adapt autonomously. For companies leading with AI or are an “AI-first company,” most of their products incorporate AI. Example: Gmail’s “Smart Compose” auto-fill capabilities that predict what you may want to write next.
Machine Learning: A specific branch of AI that consists of algorithms that can learn from data without using a complex set of rules. The algorithm’s performance improves over time as you feed it more data. AutoML helps developers with limited machine learning expertise to train high-quality models specific to their business needs. Example: Image identification applications such as Google Lens.
Deep Learning: The most specific and sophisticated use of AI. It is a subset of machine learning in which multilayered neural networks learn from vast amounts of data. Example: Google Assistant.
💡 TIP: Try not to be intimidated by AI terminology. When working with your engineering team, find a friend that enjoys teaching, and have them walk you through it, or ask them if you can shadow someone for a couple of hours to learn what they’re doing.
How to Deliver a Personalized Customer Experience Using AI and ML
Alison Wagonfeld: As marketers and consumers, we know when things are personalized and done well; it adds high value to the experience. We also know when marketers use customization poorly, it starts to feel invasive. To create a positive customer experience, I suggest doing a lot of testing and iterating so that you can determine where the line is and learn the difference between when customers feel known and uncomfortable.
You must ensure that you are marketing in a privacy-centric way and not using personal information without your customer’s consent. Consider how you can offer value to your customers so they would be more likely to allow you to use their information. Always be upfront about what data you collect and how your consumers can control their data. Invest in the right infrastructure to make sure you can generate actionable, high-quality insights that can help you meet and predict your customers’ needs.
David Edelman: The solar panel example was personalized, visual, immediate, and it had video, so I decided that I would bring this into the healthcare model when I started as CMO at Aetna. We created personalized onboarding videos for all new members. Once a person became a member, they received a video describing their healthcare plan and in-network urgent care centers in their neighborhood. It also detailed which of their medications were covered.
Out of all the members we sent it to, 70% watched the four-minute video all the way through. Those who watched the video used the call center less and went to the emergency room less, all because we gave them accurate and personalized information.
Four Business Problems AI and ML are Suited to Solve
By Alison Wagonfeld
1) Replacing Rule-Based Systems
It can be overwhelming to sift through thousands of inbound leads manually, but with ML, you can create a model that predicts what actions you need to take for each type of lead you generate.
Example: If you are a B2B marketer and receive a lead with “CEO” in their title, they should go to one category that might involve executive outreach, and if you get a lead from an “analyst,” they might like to receive an invite to the newest analytical training program. You could also collect signals for propensity models. For instance, you can organize data based on the person most likely to respond to a phone call or move through the sale.
2) Automating Processes
If you are a company with many complex and time-consuming processes, you can automate them for efficiency.
Example: In a company with hundreds of lending documents, you can screen and parse all of the data in different places using AI and ML rather than having somebody type the files manually (Google Cloud Lending Doc AI).
3) Understanding Unstructured Data
Data that is not organized in a predefined manner or doesn’t have a predefined data model can be grouped into common data points using AI and ML.
Example: If you have a favorite Major League Baseball player and only want to see home run highlights for that player, you can train models to take all the video imagery and sort out your preferred footage (MLB FilmRoom).
4) Personalizing Applications
Personalizing applications is when you find several data points and elements to create a custom experience.
Example: David’s solar panel advertisement is an excellent example of a personalized application done well. If you have collected customer insights, you can use that data to create impact, but use it carefully without becoming invasive.
Applying AI and Optimizing Teams for Digital Transformation
David Edelman: At Aetna, we were business-to-business, business-to-customer, and business-to-government. We had client-facing teams focused on specific lines of business, teams managing government programs (Medicare/Medicaid), and commercial teams working with employers and small businesses.
To optimize my team for digital transformation, I ensured our marketers had exposure to many different vendors and large-scale companies on the leading edge of marketing possibilities. We worked with the center of excellence, which shared capabilities like digital development, marketing operations, an in-house creative team, and a marketing technology and data sciences team.
Above all, I cannot emphasize the significance of testing and learning enough. We set up “agile pods,” which were clusters of people involved in marketing strategies, marketing operations, analytics, creative and tech, and compliance support, who were constantly testing and learning, and quickly getting things out the door. Eventually, as some of our initiatives scaled, they couldn’t be handled by regular basic marketing analysis; we had to build AI capability to drive and do massive multivariate testing.
It’s not just about having the AI, and it’s not just about standard organizational structure; it’s also about how you operate and be more agile to get the full value.
Three Helpful Tips for Using Data and AI
By Alison Wagonfeld
1) Have a Solid Data Foundation Grounded in Consumer Trust
Starting with a solid data foundation is essential because using these types of ML models at scale can produce an extreme version of “Garbage In, Garbage Out.” No matter how accurate the method is, the results will be wrong if the data is invalid. You must ensure that all the privacy elements are in place and that you are not using Personally Identifiable Information (PII).
Example: For data-rich industries like Retail (with strong loyalty programs, e-commerce, and direct-to-consumer sales), companies have likely invested in strong data taxonomy through years of marketing to consumers, but if you are in a data-poor industry where many sales go through third parties, like Consumer Packaged Goods selling through convenience stores or automotive selling through car dealers, think about the value exchange you can offer that will make your consumers willingly share data with you across their journey.
2) Use Respect and Empathy Across the Customer Journey
Treat your customer with empathy and respect and be sensitive to their interactions with you. Suppose you create opportunities to engage with customers, but it only shows up in one area; you may lose much of the brand equity that goes with having these thoughtful and personalized experiences.
Example: Let’s say you have a customer named Jane. You should be able to act on Jane’s data across all her interactions with your brand, from advertising to the call center. Jane has voluntarily given her data to you along the way, and this is how you can give your customers a seamless experience.
3) Build to scale
Graduation from Proof of Concept/Pilot has been the bane of AI/ML for a long time. While having these different opportunities to test and learn is important, don’t be afraid to start small, build something, and then figure out how to scale it more broadly. Focus on what is helpful for your company and your customer, as this will vary across industries and business models. Part of this entails figuring out what tech and talent you need to make it happen. Experiment and prove value to generate broader buy-in and always have a plan to scale.
Example: Oreo Cookies did an interesting ‘Test and Learn’, where they realized that if they market Oreos to people at different times of the day with various messages through contextual marketing, they could reach targeted audiences.
Managing Inherent Biases in Data
Alison Wagonfeld: When using AI, being aware of AI principles is essential (For reference, here are Google’s AI principles.) One example close to my heart is avoiding creating or reinforcing unfair bias. When working with datasets, make sure these are representative of the wider society, and you do not end up having an unjust impact on people due to their race, nationality, gender, income, etc.
With our Google Pixel phone, we found that historically racial bias in camera technology led to unacceptable experiences for people of color, such as over-brightening or unnaturally desaturating skin tone in photos. Google went back to the core sets of photography used at the beginning of the process and brought in a diverse range of specialists to help significantly increase the number of portraits of people of color in the data that trains our camera models. This adjustment created a more equitable experience across all of our camera and image products resulting in the award-winning Real Tone technology.
💡 TIP: Always consider who is creating the original data sets and where the information is coming from to have better outcomes. As you build core data sets, ask yourself and your team to flex on different dimensions. Incorporate all the different types of people engaging with your brand. The people working on the models might have unconscious biases; are you asking them foundational questions? Train your team early about those elements or if you are working with agencies, ask them how they are thinking about bias early on because it’s harder to fix once the models are built.
David Edelman: As businesspeople, you have to take responsibility to ensure that you’re incorporating thoughts about bias into the core model you are developing. Before my team at Aetna unleashed our models ‘into the wild,’ we manually tried to see what it would generate in terms of predictive modeling. We noticed some things we had to change; it wasn’t perfect, and substantial biases needed attention.
______________________________________________________
Emerging Leader Forums, hosted by Nadine Dietz, are for emerging leaders who want a great challenge from inspiring speakers in an interactive setting with their peers.
Alison Wagonfeld, VP Marketing, Google Cloud, heads the marketing team at Google Cloud as VP of Marketing. She leads Google’s fast-growing cloud business, which includes Google Cloud Platform and Google Workspace (Gmail, Meet, Docs, Sheets, Drive, and Calendar). She has been leading the cloud marketing team at Google for the past six years and has helped grow the division to over $20B annual revenues. The San Francisco Business Journal has named Alison one of the “Most Influential Women in the Bay Area.” Before her time at Google, Alison served as an Operating Partner at Emergence Capital. Earlier in her career, Alison co-founded Quicken Loans at Intuit and served as Executive Director of the Harvard Business School California Research Center.
David Edelman, Senior Lecturer of Business Administration, Harvard Business School, has 30 years of experience working as Chief Marketing Officer at Aetna and CVS and building consultancy businesses in Digital and Marketing Transformation (with McKinsey, Digitas, and the Boston Consulting Group). He now teaches at the Harvard Business School and is an advisor to top executives in start-ups, Private Equity, and larger enterprises. Having driven large-scale change from both the client and client-service side, he helps CXOs shape their strategic direction, build their teams’ capabilities, and become more digitally agile. He frequently speaks at industry conferences and events and writes blogs on topics ranging from digital marketing to the Customer Decision Journey.
______________________________________________________
Marketers That Matter® is a community of top marketing executives coming together to pioneer the future of marketing, sharing real-time experiences, and solving current challenges.
Our parent company, 24 Seven, specializes in helping you find exceptional marketing and creative talent for your teams.